Hyperspectral Sensors: A Game-Changer for the Recycling Industry
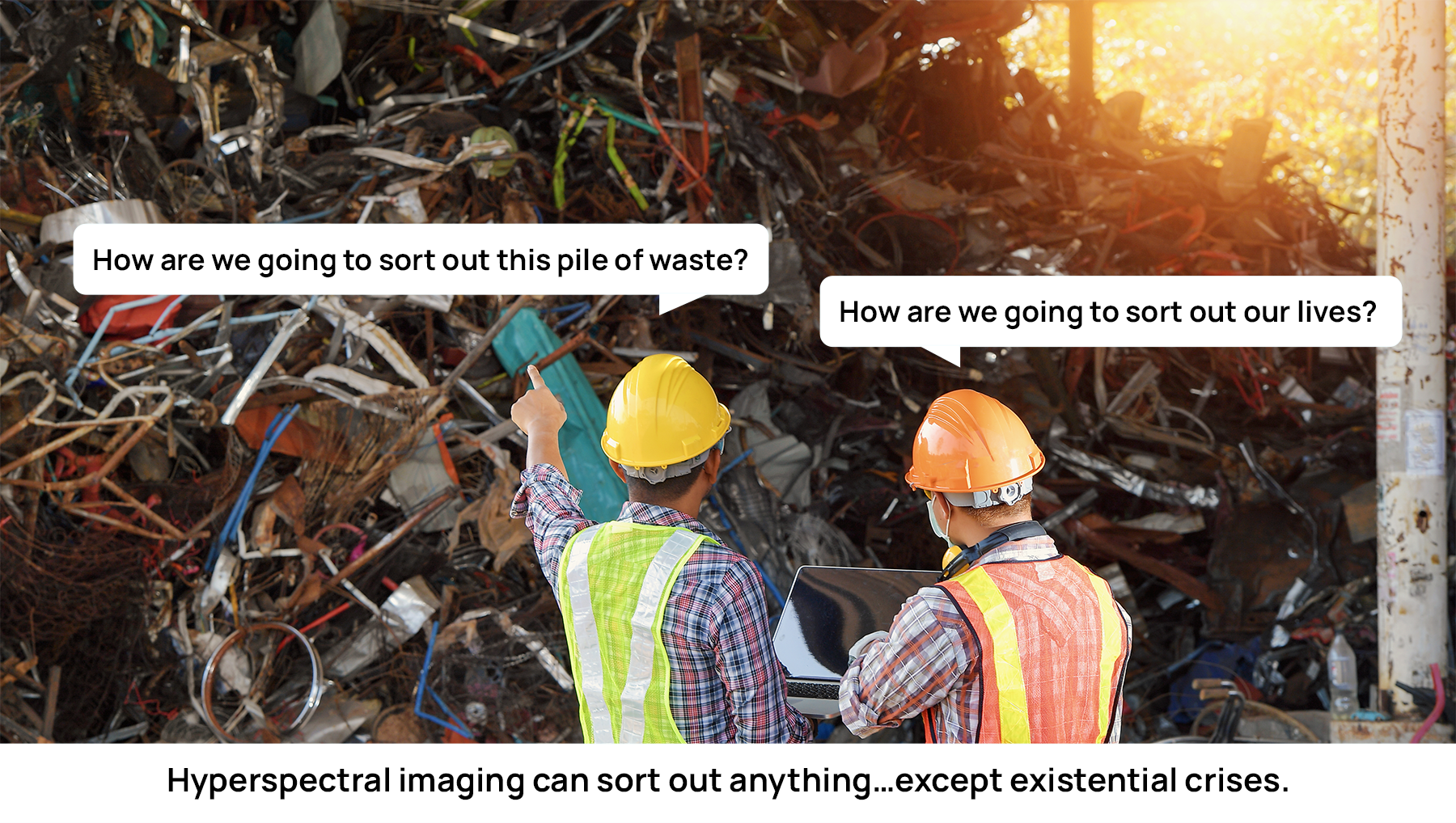
Did you know that only 9% of the plastic waste generated in the world is recycled? And that recycling one ton of plastic can save up to 16 barrels of oil and 30 cubic yards of landfill space? Recycling has become a crucial aspect of our modern society as we strive to reduce waste, conserve resources, and protect the environment. However, the recycling process is not without its challenges. One of the major hurdles recycling facilities face is the accurate identification and sorting of materials. The traditional manual sorting and visual inspection methods are time-consuming, labor-intensive, and prone to errors.
But what if there was a way to make recycling faster, cheaper, and more efficient? What if there was a technology that could automatically identify and sort materials based on their chemical and physical properties, without any human intervention? This is where hyperspectral imaging comes into play, offering a revolutionary solution to transform material identification and sorting in the recycling industry.
In this article, we dive into the world of hyperspectral imaging and its role in revolutionizing recycling. Together, we will explore the potential of this technology to enhance the efficiency and sustainability of the recycling process while reducing waste and conserving valuable resources.
The Current Challenges in Recycling
According to the latest report by the World Bank, global waste will grow by 70 percent by 2050 unless urgent action is taken. Recycling is one of the most important ways to reduce the environmental impact of waste and conserve natural resources. As the importance of recycling continues to grow, there is a pressing need for efficient material identification and sorting processes.
However, traditional recycling methods, which rely on manual sorting techniques or simple sensors, are facing significant limitations in meeting the demands of modern recycling practices.
Manual sorting is a labor-intensive and time-consuming process that relies on human visual inspection to identify and separate different types of materials. However, this approach is susceptible to human error and inconsistency. It becomes increasingly challenging when dealing with complex or mixed materials, as visual differentiation becomes difficult, leading to misclassification and contamination. Moreover, these techniques are often hazardous, as they expose workers to health and safety risks.
Accurate material identification and sorting are essential for effective recycling and waste management. Without precise identification, recyclable materials may end up in the wrong recycling streams, diminishing their value and hindering the recycling process. Moreover, the presence of contaminants can jeopardize the quality and integrity of recycled products, potentially rendering them unusable or of lower quality.
Hyperspectral imaging stands out as a groundbreaking technology that reshapes the recycling industry by addressing the challenges of traditional methods. By combining hyperspectral sensors with advanced Machine Learning and Deep Learning algorithms, it introduces a game-changing solution for accurate and streamlined material identification and sorting processes.
What is Hyperspectral Imaging and How Does It Work?
Hyperspectral imaging (HSI) is an innovative technique that combines the properties of digital imaging with those of spectroscopy. Unlike traditional imaging methods that capture only a few broad spectral bands (such as red, green, and blue), hyperspectral sensors capture detailed spectral information across a wide range of narrow spectral bands, creating a three-dimensional data cube that contains both spatial and spectral information.
Each pixel in a hyperspectral image has a spectrum that represents the reflectance or emission of the material at different wavelengths, ranging from visible to infrared. By analyzing these spectra, hyperspectral imaging can reveal the chemical and physical properties of the material, such as composition, condition, abundance, and defects.
HSI can be considered one of the best and most powerful non-destructive technologies for accurate and detailed information extraction from the acquired images, with a high level of flexibility. Here is why:
- Hyperspectral imaging captures detailed spectral information for each pixel in an image, enabling precise identification and characterization of materials with similar color or brightness but different spectral signatures, such as different types or grades of plastics, metals, paper, glass, and more.
- HSI can detect subtle variations or changes in the material properties, such as contaminants, additives, fillers, cracks, corrosion, decay, and more. This level of sensitivity allows for early detection of defects or anomalies that may impact the recycling process.
- Compared to multispectral or RGB imaging, hyperspectral imaging provides more accurate and consistent results, as it is less affected by external factors such as lighting conditions or shadows. This reliability ensures a higher level of confidence in the material identification and sorting process.
In addition to its powerful information extraction capabilities, hyperspectral sensors offer the advantage of non-destructive and non-contact analysis. They can be deployed remotely, capturing spectral data from a distance without the need for physical contact with the materials being analyzed.
However, it’s important to note that leveraging HSI requires more complex and expensive sensors and equipment compared to multispectral or RGB imaging. Additionally, hyperspectral imaging generates more data, which demands additional storage space and processing power. Data analysis and interpretation in hyperspectral imaging also pose challenges, as advanced algorithms and models are required to extract useful features and information from the spectra. Despite these considerations, the long-term benefits and increased efficiency brought about by hyperspectral sensors justify the initial investment, resulting in significant savings for recycling facilities.
How Hyperspectral Imaging Can Revolutionize Material Identification and Sorting in Recycling Processes
By harnessing the remarkable capabilities of HSI, recycling facilities can now access unparalleled accuracy and detailed insights into the materials they handle. Let’s explore the transformative applications and benefits that hyperspectral imaging brings to the forefront of recycling:
Material classification. One of the key applications of hyperspectral imaging in recycling is the classification of materials based on their spectral signatures. Each material exhibits a distinct reflectance or emission pattern across the spectrum, creating a unique spectral fingerprint. Hyperspectral sensors can capture these fine-grained spectral details, allowing for precise identification and differentiation of materials. For example, different types of plastics, such as PET, HDPE, PVC, LDPE, PP, or PS can be accurately classified, even when they have similar color or appearance. Similarly, metals, paper, glass, and other materials can be reliably identified based on spectral characteristics. This capability ensures that materials are sorted correctly, minimizing contamination and maximizing the value of recyclable resources.
Material quantification. Beyond classification, hyperspectral imaging enables the quantification of material properties, such as the concentration or purity of recyclable materials. By analyzing the spectral data, it is possible to determine the presence and amount of contaminants, additives, fillers, and other substances within the materials. This information is invaluable for assessing the quality and suitability of materials for recycling. For instance, hyperspectral imaging can identify and quantify the level of contaminants in plastic waste, such as PVC contamination in a stream of PET bottles. By accurately quantifying these impurities, recycling facilities can optimize their sorting processes and enhance the purity of recycled materials.
Material separation and recovery. Moreover, hyperspectral imaging enhances the efficiency and accuracy of material separation and recovery in recycling processes. For example, hyperspectral imaging can be integrated with optical sorting systems that use cameras and lasers to detect and separate materials based on their spectral signatures. This can increase the speed and precision of material sorting and reduce the need for manual labor. Similarly, hyperspectral imaging can be combined with magnetic or eddy current separators that use magnets or electric currents to separate metals from non-metals based on their magnetic or electrical properties. This can enhance the recovery rate and quality of metals and reduce the energy consumption of the separators. Additionally, hyperspectral imaging can aid in the recovery of valuable or rare materials, such as rare earth elements in electronic waste. By accurately identifying and isolating these materials, recycling facilities can extract maximum value and promote resource conservation.
Automating Material Identification and Sorting in Recycling Processes
Machine Learning and Deep Learning algorithms, with their exceptional predictive power, have emerged as key tools for analyzing hyperspectral images. These algorithms examine the data captured by hyperspectral imaging on a pixel-by-pixel basis to discern the composition of the object. Machine Learning and Deep Learning algorithms can be trained using extensive datasets of spectral signatures, enabling precise identification and sorting of waste materials. Through their combined capabilities, they offer a remarkable opportunity to automate material identification and sorting processes within recycling facilities.
By providing an unprecedented level of accuracy and efficiency in the identification of materials, hyperspectral imaging is able to detect contaminants like PVC ensuring that no harmful material enters the production chain during processing. It enhances purity rates in plastic packaging recycling, resulting in higher quality products and increased profits for recyclers.
In a recent study, researchers achieved an impressive 98.2% accuracy in classifying recyclable plastics from mixed waste streams using machine learning algorithms and hyperspectral imaging. By applying image segmentation and training a neural network model, automated material identification and sorting can enhance efficiency and worker safety in recycling facilities.
Hyperspectral Imaging Leads the Way
The potential impact of hyperspectral imaging on the recycling industry and society is significant. It not only boosts recycling rates and reduces waste but also contributes to resource conservation and environmental sustainability. By adopting hyperspectral imaging technology, recycling facilities can unlock a multitude of advantages, including improved operational efficiency, enhanced product quality, and reduced costs.
Are you looking to develop innovative recycling processes that maximize efficiency and environmental impact? Explore the possibilities with Softarex. Our expertise in machine learning and software development can help you integrate hyperspectral imaging solutions tailored to your specific needs. Together, we can shape the future of recycling and contribute to a more sustainable world.