Predictive Quality Analytics in Manufacturing
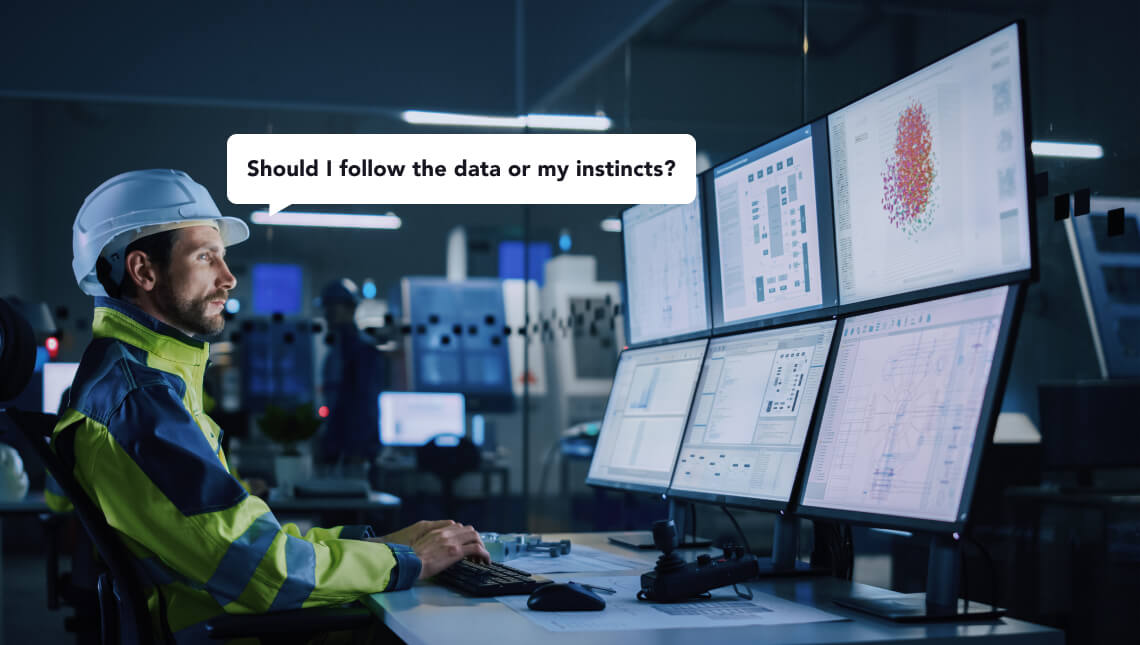
In today’s business environment, customers expect high-quality goods that are delivered quickly, and companies need to bring better products to market faster to stay ahead of the competition. For manufacturing companies, the quality of their items is especially important as it directly impacts their success in a competitive marketplace.
Historically, research shows that manufacturers that invested in technology during the years leading up to a recession observed much higher revenue growth in the recovery, following a downturn in the economy.
Roger O’Brien, Head of AMAP and Project Lead at the Sustainable Advanced Manufacturing (SAM) Project
In the present day, directing resources towards Industry 4.0 technologies, process enhancements, and skilled personnel can yield benefits in the future. Digital transformation and adoption can also help improve organizational resilience and responsiveness to customers. Moreover, manufacturers that implement process improvement technologies can anticipate immediate improvements in quality, efficiency, and productivity that can translate to substantial long-term gains.
AI-driven technology in Manufacturing
Precision manufacturing, in particular, demands a high level of accuracy and consistency to meet the strict standards set by various industries. One way to ensure this is by utilizing cutting-edge technology like artificial intelligence (AI) and machine learning (ML).
The past two difficult years in business accelerated the use of AI in systems, and organizations are now more willing to take advantage of the efficiency benefits and enhanced capabilities that AI systems offer. Deloitte’s survey on AI adoption in manufacturing revealed that 93 percent of companies consider AI as a pivotal technology to promote growth and innovation in the sector.
A new forecast from the International Data Corporation shows that global spending on AI-powered tools like predictive analytics solutions will reach nearly $118 billion in 2022 and surpass $300 billion in 2026. As companies increasingly recognize the significance of data and analytics, the manufacturing industry is also embracing the potential of AI. By adopting advanced analytical applications such as anomaly detection, real-time quality monitoring, and supply chain optimization, manufacturers are driving growth and innovation in the sector.
What is Predictive Analytics?
Predictive Analytics is a form of advanced analytics that examines incoming data or content to answer the question, “What is likely to happen?” It uses historical data, machine learning algorithms, and statistical models to make predictions about unknown future events and identify the likelihood of future outcomes based on specific variables. This emerging category of AI enables manufacturers to efficiently identify root causes and prevent losses in both quality and waste, leading to improved operational efficiency.
Predictive Quality in Manufacturing
Predictive Quality Analytics is based on analyzing conditions in the entire ecosystem to determine the probability of quality events and mitigating them prior to the occurrence. The capability of predictive quality empowers factories with three primary benefits to enhance their production process and eradicate quality control-related losses:
Predictive insights. Predictive quality can offer data-driven insights that enable manufacturers to minimize quality issues. Predictive maintenance, for example, relies on real-time sensor data from parts, components, or machines to predict possible defects and fix them before they actually occur. Being able to stop or adjust a process earlier can greatly reduce or even eliminate material waste or rework. In contrast, preventive maintenance typically involves daily routine checks to assess the current condition of equipment. This approach, while helpful in ensuring that machines are running smoothly, doesn’t offer the same level of proactive problem-solving that predictive maintenance does.
Automated root cause analysis. Manufacturers can benefit from predictive quality, which utilizes intelligent tools to detect underlying causes that were previously unidentified. Furthermore, it constantly evaluates vast amounts of data, providing manufacturers with precise root cause analysis for any issue. By reducing manual effort and enabling targeted investigations, predictive quality streamlines the problem-solving process for manufacturers.
Reports, real-time alerts and notifications. With the ability to integrate various data sources, predictive models enable efficient data mining and generate user-friendly evaluations that can be easily converted into readable reports for end-users and managers. In addition, predictive quality empowers manufacturing teams with real-time alerts and notifications that immediately flag any process inefficiencies, allowing for timely corrective actions to prevent issues from occurring.
Wisely used, Predictive Quality Analytics can thus lead to massive savings in the warranty costs area and help companies take a significant step towards becoming a leader in quality management.
Predictive Quality Analytics: three-step approach
Predictive Quality enables companies to make data-driven predictions of product quality. However, integrating data from different sources can be one of the most significant challenges. Here’s a three-step approach to leverage this technology effectively.
Step 1. Collect and pre-process data from key sources. When deploying a predictive analytics solution, firstly collect data from machines and sensors and integrate this data with live production data from MES and ERP systems, and offline quality data. After that, cleaning, merging, formatting, and structuring in the cloud takes place. For example, if one machine notes down the temperature in Fahrenheit and another machine takes the temperature in Celsius, then the temperature needs to be converted into a combined metric.
Step 2. Create and validate predictive models. Based on historical data, machine learning algorithms can find out the behavioral patterns that have earlier led to problems. Relationships between key process variables are determined to build a predictive quality model. When models are deployed in a production environment, they look for patterns or sets of conditions that provide early indications of inefficiencies, scrap rates or quality failures.
Step 3. Notify teams. If the real-time event starts to pursue one of those problem patterns, then the system can predict the potential result and alert factory managers. Once operators, engineers, or supervisors are notified of potential quality failures with sufficient lead time, they can take corrective action in advance to avoid producing defective products for an extended period of time.
The benefits of Predictive Quality technology and management
Prevent Quality Failures. Real-time alerts and notifications allow manufacturers to adjust processes accordingly as soon as possible. This approach enables operators to optimize first-pass yield, and remain compliant with industry standards.
Optimize Material Usage. Predictive quality alerts supervisors when desired scrap rates are about to be exceeded, thereby allowing them to adjust their processes and prevent further material wastage.By doing so, businesses can save a significant amount of money while reducing their environmental impact.
Quickly Isolate Defects. By pinpointing the specific area where a defect occurred, manufacturers can be more efficient in their efforts to limit the overall number of pieces scrapped. This helps reduce material waste and, in turn, increases productivity within the manufacturing process.
The bottom line
Few industries can benefit from predictive analytics more than the manufacturing sector. The sector generates enormous amounts of data, involves repetitive manual tasks, and presents multi-dimensional problems beyond the scope of many conventional tools. Whether it is improving quality, reducing downtime, or optimizing efficiency, AI-driven predictive analytics is the perfect technique to solve many complex manufacturing problems. This makes manufacturers more resilient and responsive to the needs of customers, helping them build loyalty and gain market share.
By partnering with the right experts, you can effectively implement predictive analysis technology into your manufacturing processes. Softarex can help you achieve this competitive advantage and embrace the full potential of AI. Start a conversation with us today to learn more.